How Smart Technologies Can Revolutionize Energy Grids
In 2024, energy management represents one of the critical challenges for the future of our economies. Beyond the headlines about decarbonization and various environmental targets to meet, we face tangible problems: outdated grids, growing energy demand, and the need to increasingly integrate renewable sources into our infrastructure. The good news? Smart technologies can solve many of these issues, transforming energy grids into efficient and sustainable systems.
Let’s explore the main challenges and how our software solutions can enhance energy management.
The Relevance of Infrastructure and the Key Role of the “Prosumer”
Traditional energy grids, designed for centralized management and primarily powered by large fossil fuel plants, no longer meet the modern needs of fluctuating demand. They require a hybrid approach that integrates renewable sources, storage systems, and local production.
A key aspect of modern energy management is the active involvement of consumers, who can become “prosumers”—both producers and consumers of energy. By installing solar panels on rooftops or investing in storage technologies, citizens can actively contribute to local energy production. This reduces the strain on central grids, minimizes energy losses from long-distance transmission, and increases the flexibility of the overall energy system.
Key Insights Presented at EUPVSEC
Just last week, i-EM presented “Optimization analysis for the best sizing and operation of photovoltaic generators in distributed electricity systems” in Vienna at EUPVSEC, the 41st edition of the international conference dedicated to research, technologies, and applications in photovoltaics.
This presentation highlighted a solution aimed at improving the effectiveness of photovoltaic installations in a distributed generation scenario, providing valuable information to prosumers and planners for identifying the optimal grid design. The goal is to reduce financial risk through more accurate strategic planning, enabling reliable microgrid sizing that leads to investment savings, minimizes the risk of microgrid under or over performance, and prevents poor-quality service.
But the study doesn’t stop there. As we know, new technologies like Artificial Intelligence (AI) and Machine Learning are opening new frontiers in energy management. Advanced algorithms can predict future demand, optimize production, and improve the operational efficiency of grids.
In fact, i-EM’s solution offers an optimization analysis to find the best scheduling of power flows for the following days (up to 7 days ahead) by exploiting ML-based forecasting algorithm for the load, PV generation and electricity price profiles, used in combination with a mixed-integer programming (MIP) optimization approach to find the best scheduling. It is also applicable to existing systems that include energy storage solutions.
This study has already been practically applied in ‘behind-the-meter’ scenarios for industrial and commercial settings, as well as in off-grid systems in rural areas of India. The performance and accuracy of the ‘best design’ solution were evaluated by comparing the payback time indicated by the solution with other sources of information, such as PV plant installers. According to feedback from end users, based on their experience in real scenarios, the discrepancies in the payback time value were less than 10% in terms of months. Regarding the ‘optimized scheduling’ solution, we compared the economic benefits against the strategies commonly used to manage power flows in systems that include generation, local consumption, and storage systems. In this case, the estimated economic benefits ranged between 5% and 10%.
Key items for Optimizing Photovoltaic Systems for Maximum Efficiency and Profitability
Let’s now look at a solution to support PV plant designers in identifying the best system configurations and optimizing energy flows for operational systems, leveraging satellite data, statistical models, and predictive analytics.
Optimal Sizing:
- PV Resource Analysis: Satellite data is used to assess the solar resource at the site, with time-series data processed over at least one year.
- Electric Consumption Profile: Load profiles are determined using archived consumption data with a granularity of 15 minutes or 1 hour, accounting for seasonal variations.
- Additional Components: For systems with energy storage or electric vehicle charging, parameters like storage capacity and installation costs are defined.
- Objective Function: The goal may focus on minimizing Pay-Back Time or maximizing grid independence, factoring in storage system performance (e.g., cycles, discharge depth).
Simulation and Analysis:
- Scenario Simulations: Multiple scenarios are simulated, altering PV and storage sizes to assess energy balances, where over-production feeds the grid, and storage charges or discharges based on demand.
- Performance Metrics: Key indicators such as self-sufficiency and financial benefits are calculated to identify the best system design.
Energy Flow Optimization:
- Load and Generation Forecasting: Statistical models predict load profiles, solar generation, and energy prices, with forecasts having a time granularity of 15 minutes to 1 hour.
- Optimization via MIP: A Mixed-Integer Programming approach solves the optimization problem, setting constraints for power flow, storage interaction, and economic benefits from grid interactions.
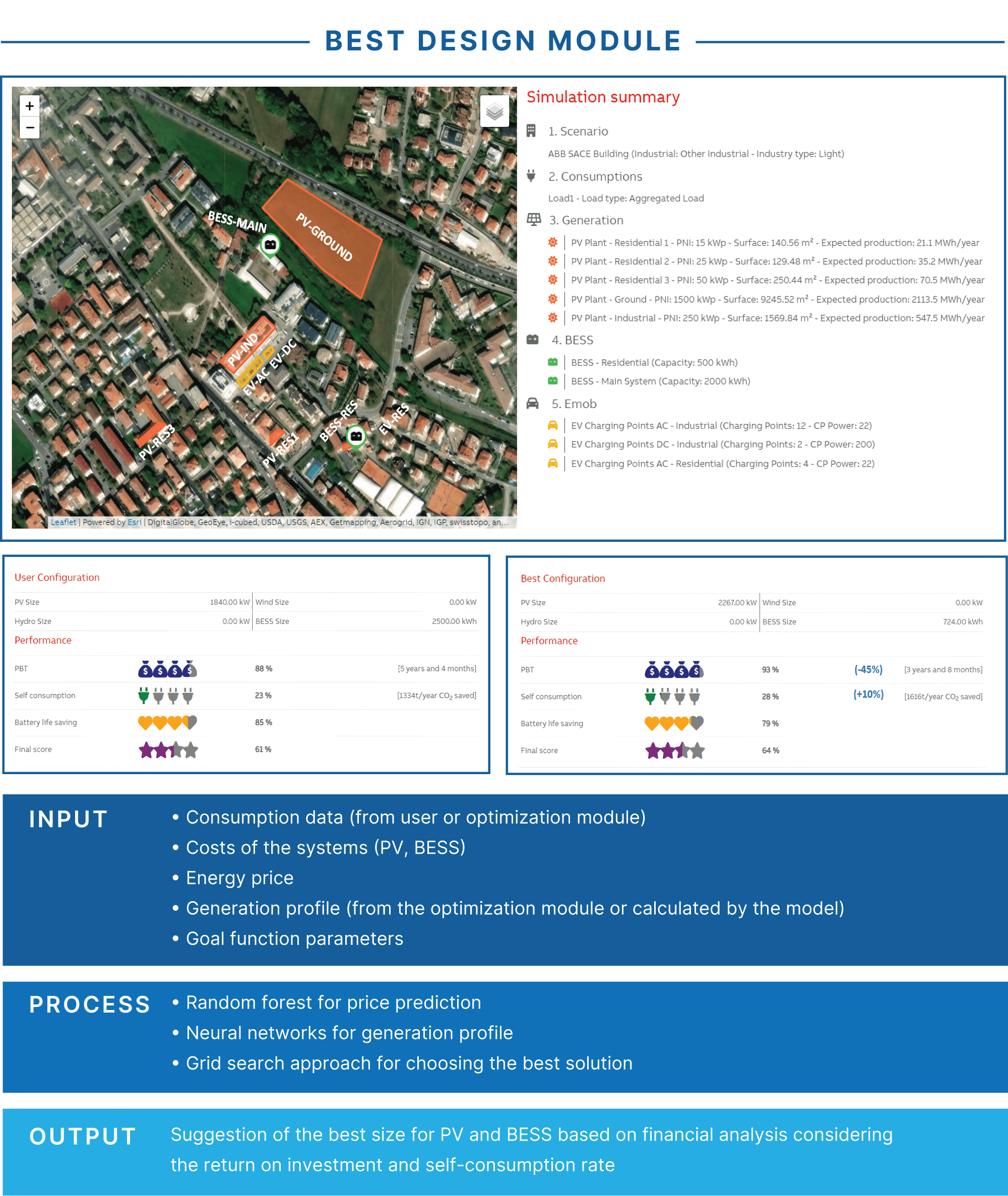
An example of our approach with our solution X-EM
Why Our Approach is Pioneering the Future of Photovoltaic Optimization
The true innovation behind this approach lies in the integration of different data sources, processed through advanced statistical models. By combining satellite Earth-Observation data with machine learning algorithms, this solution can simulate the behaviour of distributed generation systems, accurately predict key management variables—such as energy production, consumption, and electricity prices—and optimize energy exchanges with the external grid.
What sets this solution apart is its global reach. With satellite data, it can assess photovoltaic potential in any location, enabling highly customizable simulations for PV systems in different configurations. When combined with Numerical Weather Prediction (NWP) data, calendar information, and historical archives, this data fuels powerful machine learning models to forecast energy consumption and production patterns with precision.
The scientific impact of this work is significant because it accelerates and simplifies the integration of distributed photovoltaic systems into the wider power grid. Its flexibility allows it to be applied to a variety of scenarios. Whether it’s for “behind-the-meter” applications where individual users can optimize their own energy goals, for energy communities integrating multiple systems, or even for off-grid electrification in rural areas, this approach offers an adaptable, scalable solution.
Moreover, this system isn’t limited to solar power, it can integrate wind, hydroelectric resources, and multiple storage technologies, such as electrochemical batteries or Pumped Thermal Energy Storage (PTES). This opens new possibilities for maximizing the efficiency of renewable energy systems by combining diverse energy sources for optimal results.
This mix of scientific accuracy, technological versatility, and practical application ensures that this solution is at the forefront of energy innovation.
Conclusion
Tailored Energy Optimization: The Future of Photovoltaics is here
The results of our innovative approach are already proving effective in real-world applications. Our “best-design” solution is being implemented globally in industrial and commercial settings to maximize the efficiency of photovoltaic installations, improving energy self-sufficiency and boosting revenue from grid exchanges. In off-grid scenarios, such as in rural India, the solution is helping reduce reliance on conventional generators and improving community living standards through sustainable electrification.
Some feedback from the users
- Accuracy in Payback Time Calculations: less than 10% variation compared to traditional tools, while offering greater flexibility and customization.
- Economic Benefits of Optimization: estimated between 5% and 10% compared to non-optimized systems, attracting interest from major operators in the PV and storage industries.
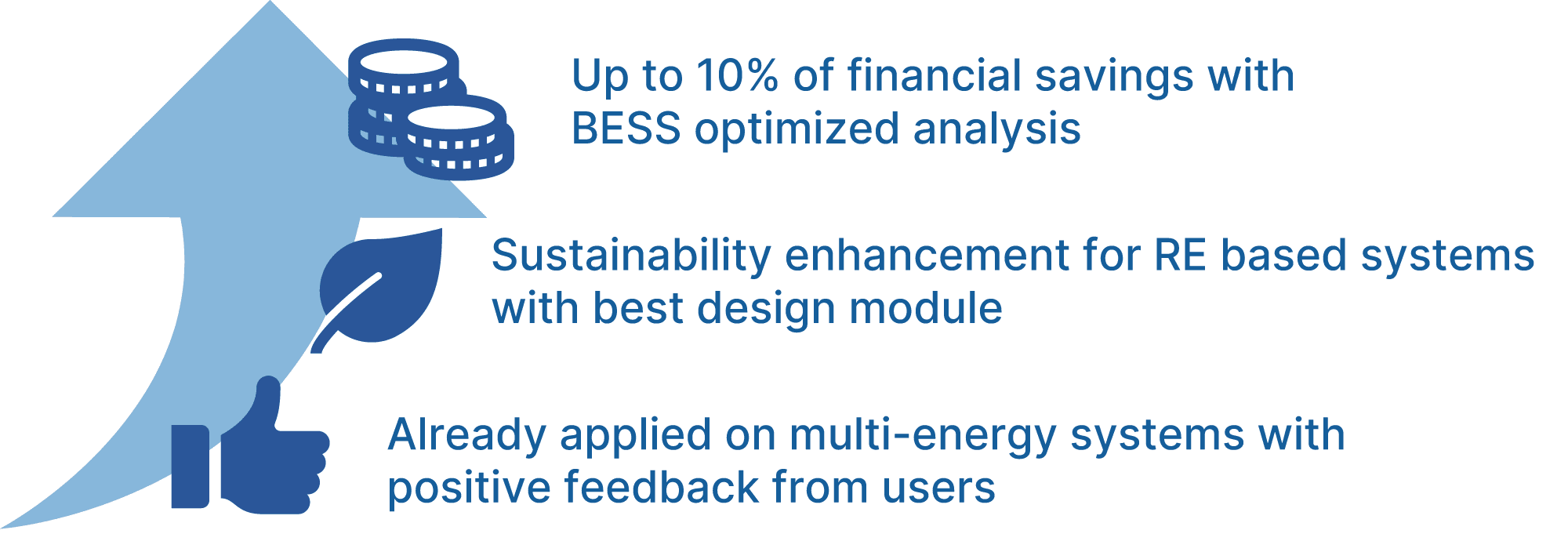
The versatility of our solutions, available as easy-to-use SaaS, allows users to tailor simulations to their specific needs. This adaptability, combined with the potential to integrate multiple renewable sources, makes our approach a game-changer for accelerating renewable energy integration.
Don’t let these words remain just a simple read; transform everything you do into energy and action.